The ever-innovative chemical industry is constantly on the lookout for new substances and chemical products with novel properties.
In 2017 alone, the industry spent more than $45 billion on research, but how much of this money was wisely invested?
The fact is a large proportion of R&D investment never yields a return but is instead used up on a ‘blind alley’ of an idea from a chemistry researcher.
However, this is a process that is about to change, because computer scientists have now teamed up with chemists from Princeton University to create an artificial intelligence that can problem-solve organic synthesis.
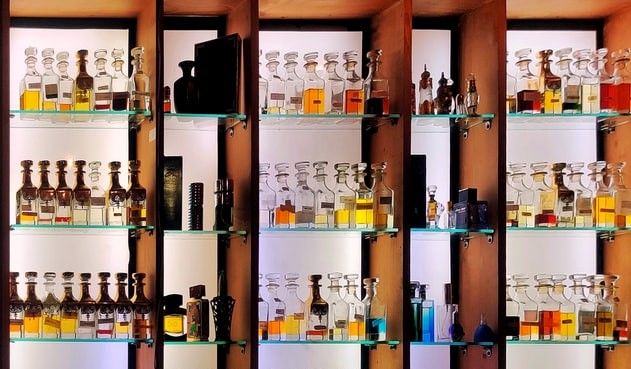
The results of the cooperation have now been published in the journal Nature, where the research team outlines their open-source software which provides a state-of-the-art optimization algorithm.
As the Princeton press release explains, the algorithm is, “… based on the Bayes Theorem, a mathematical formula for determining conditional probability. Bayesian optimization is a widely used strategy [which] allows people and computers to use prior knowledge to inform and optimize future decisions.”
By adapting this theory with machine learning now allows for faster and more efficient syntheses of chemicals.
“Reaction optimization is ubiquitous in chemical synthesis, both in academia and across the chemical industry,” said Prof. Abigail Doyle who co-wrote the study. “Since chemical space is so large, it is impossible for chemists to evaluate the entirety of a reaction space experimentally. We wanted to develop and assess Bayesian optimization as a tool for synthetic chemistry given its success for related optimization problems in the sciences.”
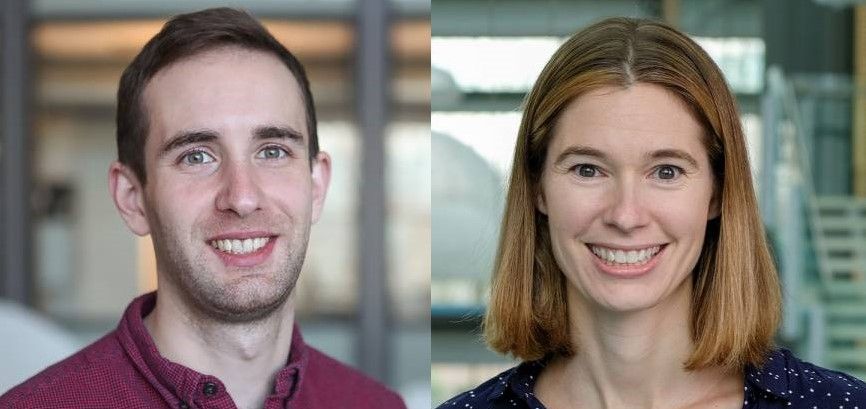
“I come from a synthetic chemistry background, so I definitely appreciate that synthetic chemists are pretty good at tackling these problems on their own,” notes Benjamin Shields, a postdoctoral researcher and the study’s lead author. “Where I think the real strength of Bayesian Optimization comes in is that it allows us to model these high-dimensional problems and capture trends that we may not see in the data ourselves, so it can process the data a lot better.”
The use of AI also avoids false human assumptions and biases.
“Users start by defining a search space—plausible experiments to consider—such as a list of catalysts, reagents, ligands, solvents, temperatures, and concentrations,” explains the scientific journal Phys.org. “Once that space is prepared and the user defines how many experiments to run, the software chooses initial experimental conditions to be evaluated. Then it suggests new experiments to run, iterating through a smaller and smaller cast of choices until the reaction is optimized.”
“In designing the software, I tried to include ways for people to kind of inject what they know about a reaction,” Shields said. “No matter how you use this or machine learning in general, there's always going to be a case where human expertise is valuable.”
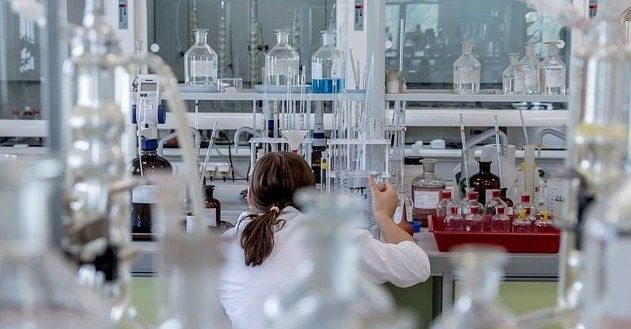
Crucially, the use of AI reduces research costs and gets to a successful chemical reaction and product much faster. It is a scientific advance that could save chemical companies millions of dollars.
But perhaps even more importantly, is that the process allows the AI to take routes that human researchers might have dismissed out of hand. In this way, the AI is able to imagine chemical products that chemists would never have considered.
“Reaction optimization can be an expensive and time-consuming process,” explains Ryan Adams, a professor of computer science who played a key role in the breakthrough. “This approach not only accelerates it using state-of-the-art techniques, but also finds better solutions than humans would typically identify.”
Photo credit: Omkar Jadhav on Unsplash, Princeton University, Michal Jarmoluk from Pixabay, & David Mark