All the experts agree: Artificial Intelligence is the future of business and nowhere is this more true than in the chemical industry. It is a sector of the economy whose intricate processes, complex research programs, and convoluted supply chains are in a never-ending search for efficiency and innovation.
But where is all the AI?
A recent survey of the issue conducted by IBM found that, “Eighty per cent of chemical industry executives … say that AI will be important for the success of their business over the next three years.”
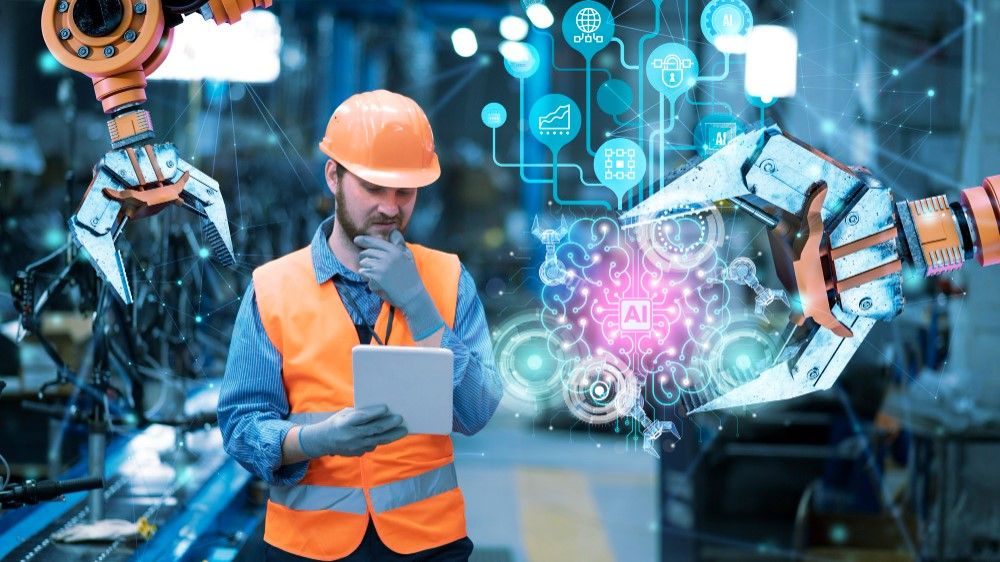
That makes perfect sense. Industrial chemical production processes, the procurement of chemical feedstocks, international supply routes, and the time-consuming development of new formulas are all data heavy and ideal for the application of AI. So why is it that the same IBM study also found that six out of every ten chemical companies polled had yet to begin an AI implementation strategy?
The reasons for a lack of action towards AI in chemical business are manifold, but here are the main sticking points.
A Lack of Know-How
Like any new technological field, finding experts can be challenging, particularly in a specialist sector such as the chemical industry. All too often, a key obstacle to the development and application of AI in chemistry is the lack of people with the interdisciplinary abilities necessary to combine competence in computer science, data science, and industrial chemistry.
Constructing AI Systems is Expensive and Time-Consuming
Most teams in a chemical company are already overworked and struggling to meet strict deadlines and targets. This can make it challenging to allocate resources to installing AI solutions, especially if they are disruptive to current systems that are operating at full capacity. Additionally, the impact of an active AI system may take months before noticeable results are seen.
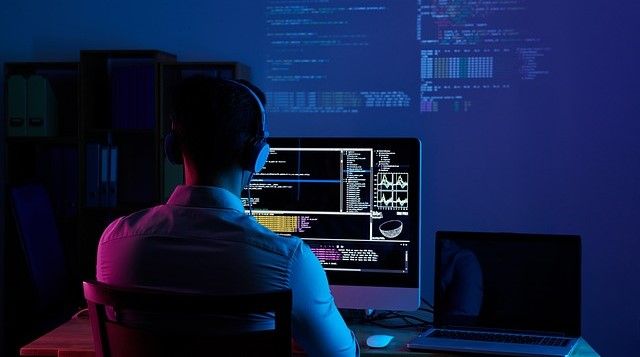
The Promotion of AI is Often Led by Technical Teams
While the advantages of AI systems in a chemical company are widely unknown, the most informed personnel are typically those working in technical departments. Any suggestions from them to implement new systems, particularly those handling sensitive data and client lists, or for letting ‘machines’ become involved in decision making are frequently viewed with suspicion from other departments.
Consequently, gaining organisation-wide buy-in for an AI initiative is likely to meet resistance from procurement teams, sales staff, logistics management, and those on the factory floor who may well see AI as a hinderance rather than a useful tool.
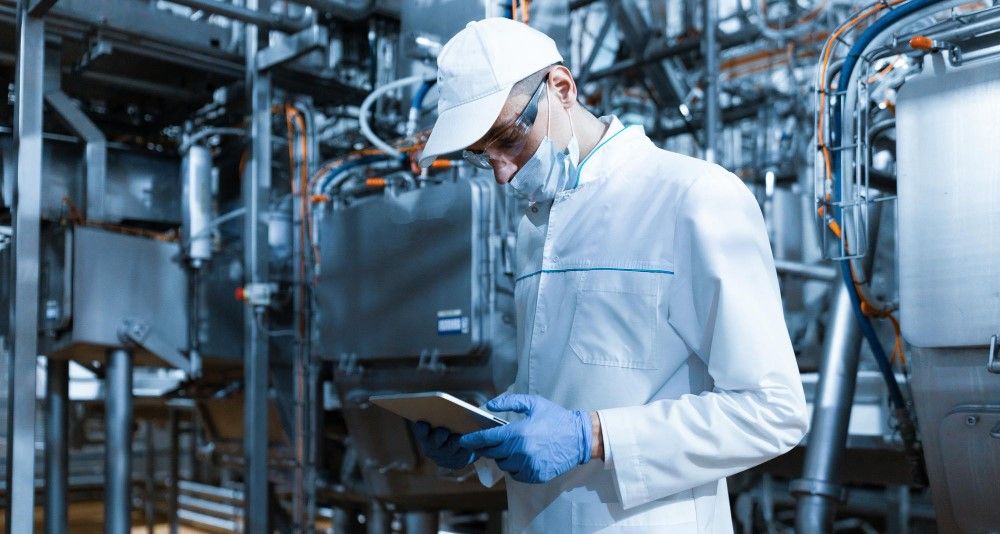
However, if these issues can be overcome, then those chemical businesses taking the first step into AI implementation will find quick rewards in many areas of a chemical business, because where it has been applied, AI is already paying for itself through cost savings and efficiencies.
“Artificial intelligence (AI) has proven to be an effective tool in optimising operations for chemical factories to meet sustainability targets, particularly in reducing energy consumption, including electricity usage,” explains Benson Wang, a Product Director for Profet AI, an AutoML enterprise solution for the manufacturing sector. “This is done by using data such as historical electricity consumption, environmental conditions (temperature and humidity), production information, and equipment operation data. Commonly we have observed that AI adoption can help chemical companies in Taiwan reduce their electricity consumption by around 3%.”
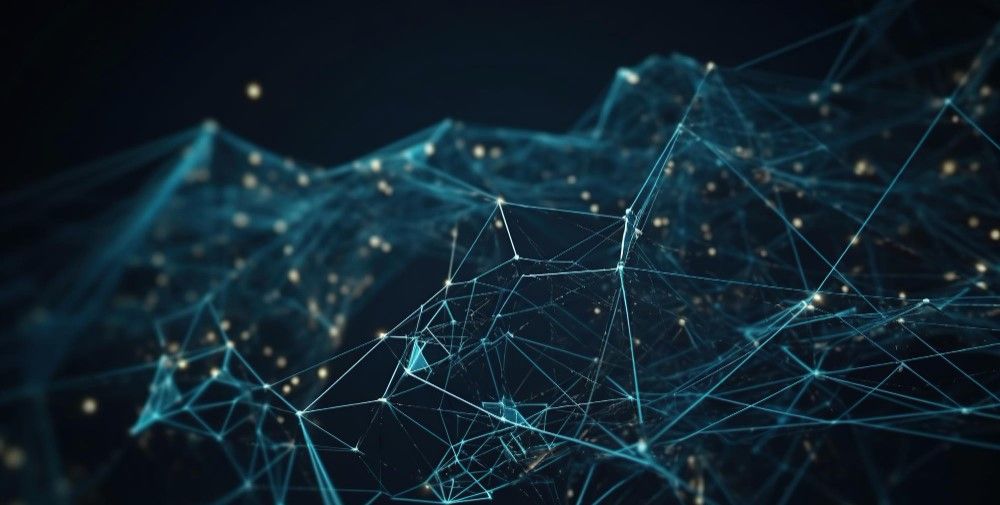
But AI already has a track record of improving other areas of the chemical industry, such as:
Chemical Industry Procurement
A recent report by the UK-based Chemical Industry Journal found that, “Raw materials costs account for 50-70% of chemical companies’ sales revenue, [yet] … many manufacturers tend to make procurement decisions based on key staff’s personal judgment or – if they do utilise data – it tends to be centred on historical trend averages for material price.”
Getting the best price and quality is vital for procurement teams and directly impacts a chemical company’s bottom line. AI can provide purchasing managers with a more comprehensive view of the entire chemical trading scenario, allowing them to make more informed pricing decisions. This is especially true for complicated chemical supply chains with distributors, merchants, and manufacturers, spread over several locations.
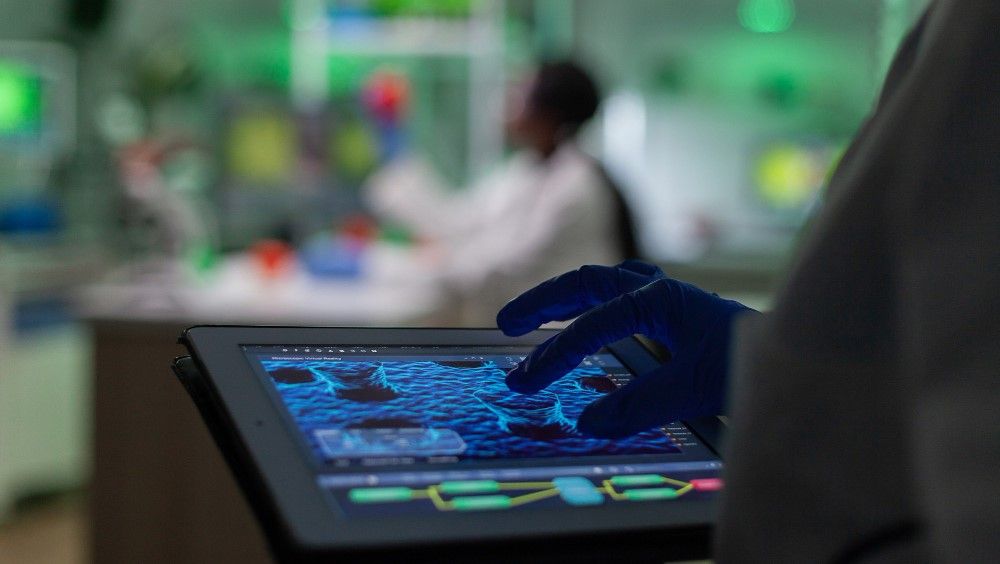
By using computer models which assess a variety of factors, such as exchange rates, market volatility, behavioural science, geopolitical events, crude oil futures pricing, and plastic market data, predictive analytics can provide purchasers with a much better picture. This makes it possible for managers to make decisions more proactively than reactively.
Industrial Chemical Research with AI and Self-Driving Labs
The long and complex process of drug and chemical formulation will be greatly shortened and simplified with the application of AI.
One example of how this will happen is through the creation of ‘self-driving labs’ - cutting-edge test grounds that seamlessly combine technology, robotics, and artificial intelligence.
As a World Economic Forum report states, “The advent of Self-Driving Labs (SDLabs) represents a transformative leap in research and development (R&D) that is reshaping our understanding of time efficiency and of what’s possible. As industries grapple with challenges related to energy, sustainability and health concerns, along with productivity declines and the need for faster innovation, SDLabs emerge as a pivotal solution and a transformational opportunity.”
Quality Control of Chemical Products with AI
When a production line malfunctions in the chemical business, it is imperative to act quickly as batches can easily become contaminated and spoil. Despite this, the standard approach for resolving quality concerns in the chemical production is to rely on employee expertise and a trial-and-error approach. These inefficiencies can be removed by combining sensors, computer vision technologies, and AI-based tools. Not only does this enable the prompt identification and resolution of problems, but the AI can also learn from mistakes in the process and so advise of how to avert similar ones in the future.
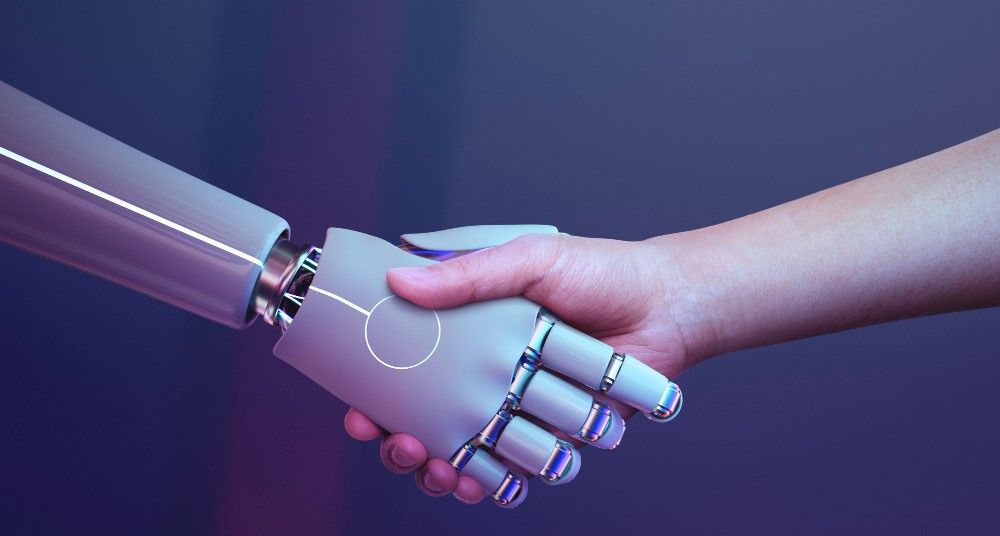
“We worked with one client specialising in cellulose nanofiber coatings, which uses roll-to-roll processing in which films or soft boards are unrolled from cylindrical rolls,” recalls Wang. “This is a complex process with a relatively high defect rate of 12%. AI-powered quality factor analysis allowed them to quickly resolve defect issues, reducing the defect rate to only 5%.”
Although concerns over the cost and resource application for installing AI are real, chemical businesses can gain substantial advantages in terms of long-term cost savings, increased productivity, enhanced quality of products, or the accelerated creation of new products.
But only if they are ready to take the leap.
Photo credit: Rawpixel on Freepik, Innova labs from Pixabay, Rawpixel, ojosujono96, usertrmk, DC studio, & Freepik