There is plenty of hype, there is plenty of investment, and there is plenty of activity on how AI is coming and what it will change.
But how is it really being applied in the chemical industry and what are the greatest benefits?
Despite the complexity of industrial chemical processes, the simple answer is in energy savings which are not only lowering costs but are also helping chemical manufacturers reduce carbon emissions.
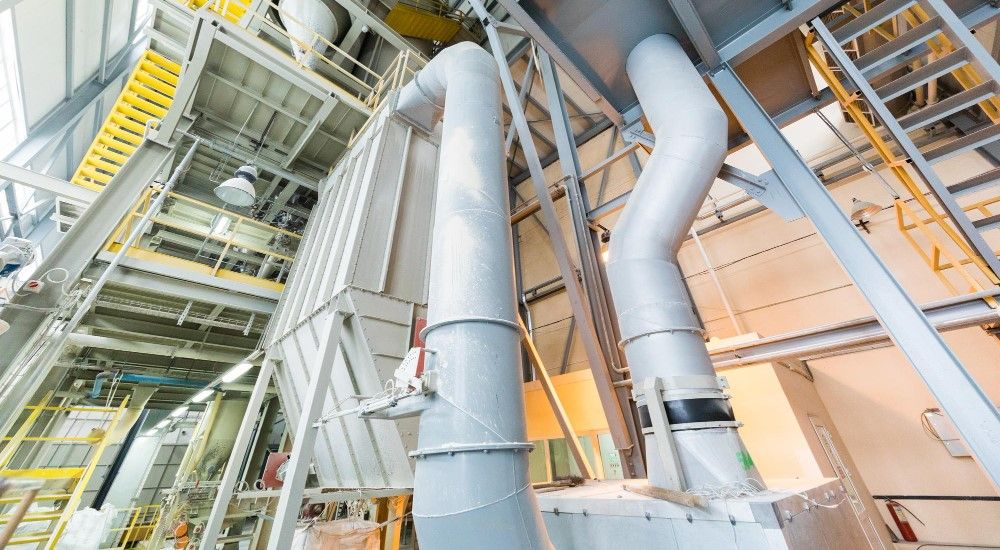
“The transformative impact of Artificial Intelligence (AI) technology in the chemical industry is enabling significant energy efficiency improvements of 20% to 40%,” writes Rohit Kochar, a business analyst with 25 years of industry experience, who is the current CEO of Bert Labs.
For this reason, building a digital transformation infrastructure and integrating AI into daily operations (while expensive) are imperative for chemical firms.
In the past, technological innovation has been paramount in the chemical industry, with processing advantages adopted to improve production control, safety, and productivity. However, adoption of the latest and greatest innovation; AI, has been surprisingly slow. This is especially true in Europe, given that soaring energy costs, increased competition from Asia, feedstock advantages in the US and Middle East and dwindling profit margins, have put chemical companies such as BASF and INEOS under extreme pressure in their traditional markets.
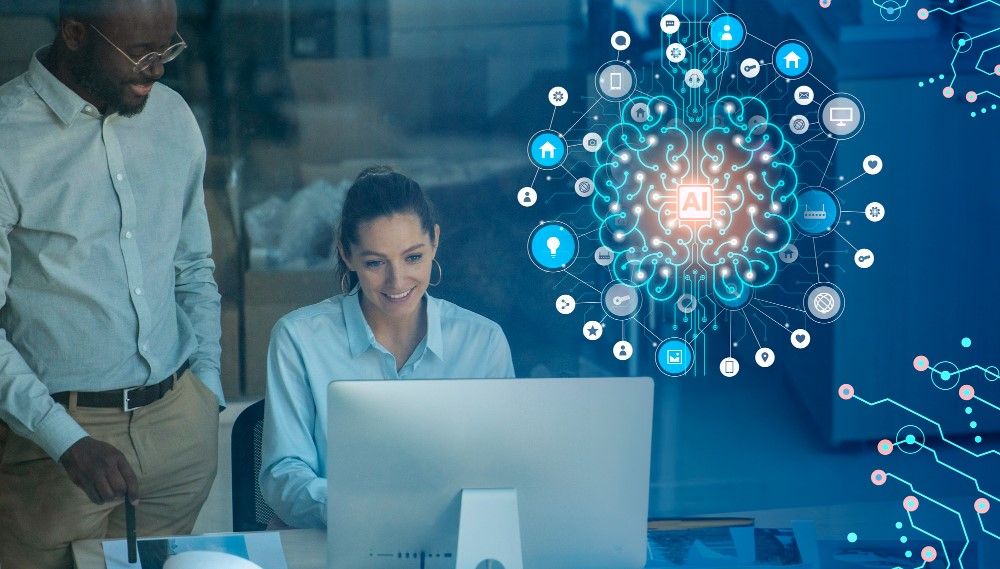
“Most chemical companies have some level of automation,” observes Kochar, a former Managing Director at Hays PLC, Vice President at American Express, and Vice President at General Electric. “They have a robust DCS system, that can capture the process data from the plant and can also execute controls in the field including safety control actions. The industry need is of an AI Technology that can build on top of this infrastructure and start giving benefits immediately on deployment.”
The foundation for a Digital Transformation infrastructure is based on the following three principles:
· Sensing - real time capture of high granularity, high coverage, high quality, high variety, and high volume of controllable data points of operational and energy consuming processes, as well as overall process, production, and quality data.
· Connection - real time transmission of upstream and downstream data.
· Action - real time command of the process conducted with ongoing data collection and the generation of analysis and data representation for human control, such as energy dashboards and visual analytics.
As a self-learning tool, the AI computer model will continually update itself, constantly refining its decision-making to reduce costs and lower emissions. This means that early adopters of AI stand to gain more and will be well-positioned to benefit in the fiercely competitive chemicals sector.
How AI is Connected to a Chemical Facility
In order to deploy AI solutions in a chemical plant, a typical plan of action involves having AI and chemical experts assessing the facility examining the plant's current data capturing system, data storage policy, and installed process controllers (PLCs), as well as its control system architecture and process constraints. The baseline data to be evaluated will be energy use, production, yields, and maintenance expenses, from which a list of the chemical plant's digital transformation infrastructure requirements will then be drawn up.
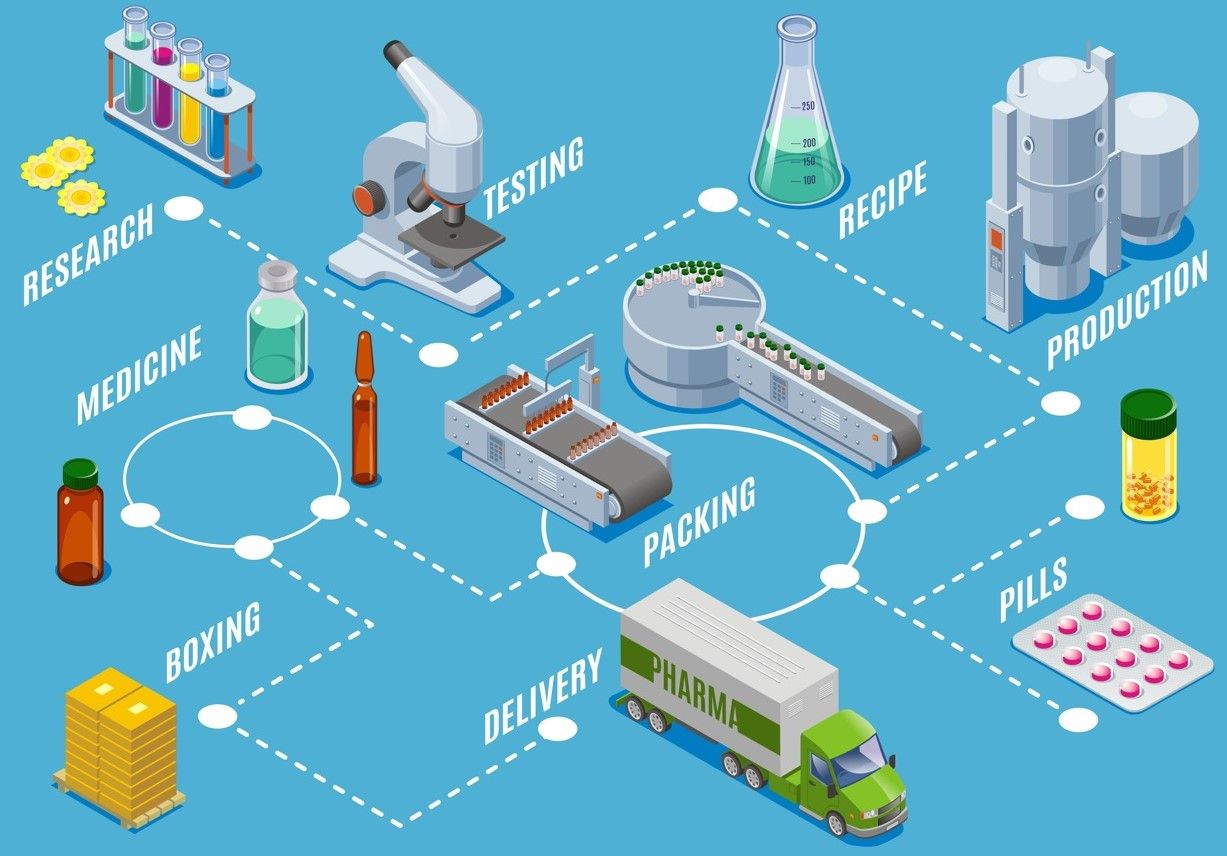
This will include calculating the number of IoT devices, the wireless network architecture plan, and a data management software platform held in either the cloud or a local server.
Chemical-based modelling is done from the plant’s historical operational and process data using design data, fundamental principles, and deep learning neural network modelling. This produces an operational digital twin of the chemical facility, which simulates with an accuracy of more than 95%, the actual plant environment. Once they have been validated and tested, deep reinforcement learning agents are employed to increase energy and production efficiency.
Case Study - How AI is Employed in The Electrolysis Process
Chemical companies are always searching for ways to reduce the power costs associated with an electrolysis plant because they are energy-hungry machines. Yet energy efficiency can be achieved with AI technology without inhibiting operations.
As Kochar explains, “Digital Twin of the Electrolysis process can give accurate predictions for the in process and output parameters like chlorine & hydrogen pressures, caustic flow rates and concentration, differential pressure across ion exchange membrane. The Reinforcement Learning Agent Optimization Algorithm will use the predicted values and give the best combination of control parameters like voltage applied, brine flow rate, brine temperature to bring power reduction for the same output values.” Noting that, “The results are savings of at least 30% in power consumption.”
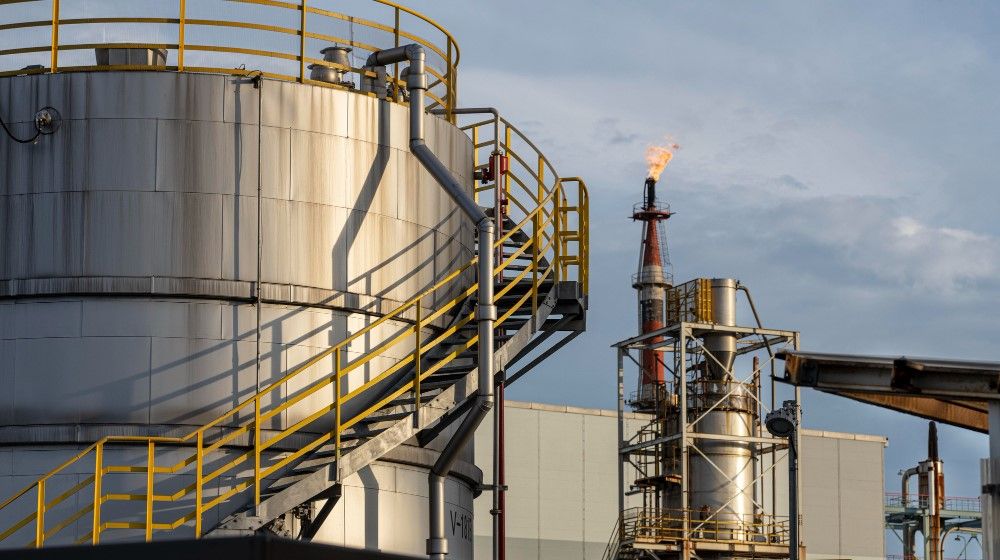
Case Study - How AI is Employed in an Ammonia Recovery Plant
The easiest efficiencies to be made in an ammonia recovery plant come from reducing steam consumption in a complex process involving both low and medium pressure steam. Making optimum savings is difficult for a human, but an ideal task for AI.
In this situation, a Digital Twin of the ammonia process can give accurate predictions for the in process and output parameters, such as the quantity of ammonia recovered, the column temperature and pressure, and the level of ammonia in the column bottom. From this, a Reinforcement Learning Agent Optimization Algorithm is used to predict values which give the best combination of control parameters, such as medium and low-pressure Steam flow, cooling water flow, and lime addition to regulate steam reduction. If performed correctly, AI can save such a facility as much as 40% of steam consumption.
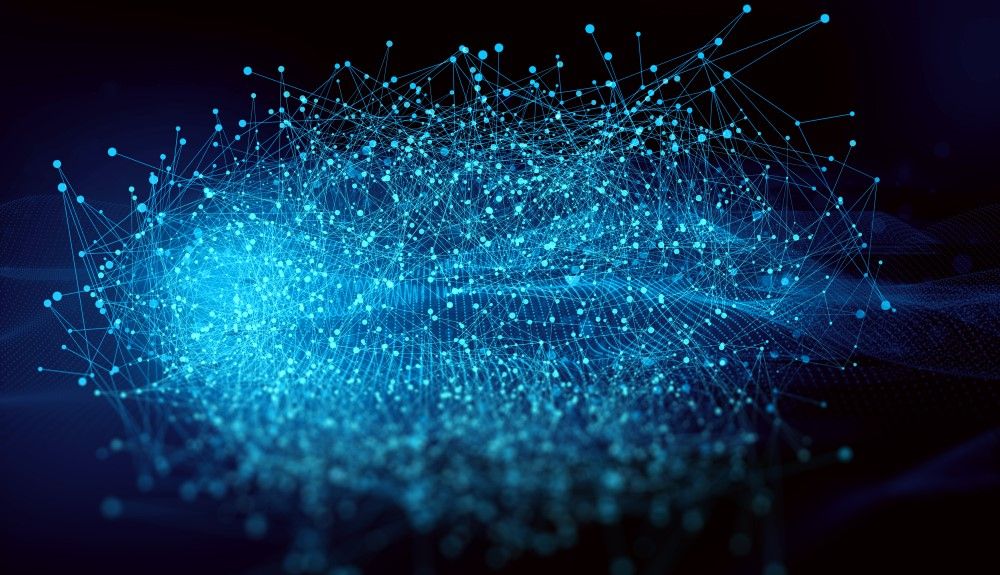
AI offers numerous advantages for the chemical industry in optimizing chemical processes, improving safety, boosting research speed, and aiding quality control. Each of these benefits alone are ground-breaking for the business of making and supplying industrial chemicals. However, the ability to streamline processes to reduce energy consumption, and thus reduce costs and lower carbon emissions is truly revolutionary.
The result is that rather than being an additional cost to industrial chemical production and supply, it actually provides essential cost savings.
Read more on this at What Will a Fully Digitalised Chemical Industry Look Like? or Where is the AI in Chemical Manufacturing and Supply?
Photo credit: Macrovector on Freepik, Freepik, Usertrmk, kjpargeter, upklyak, & Freepik